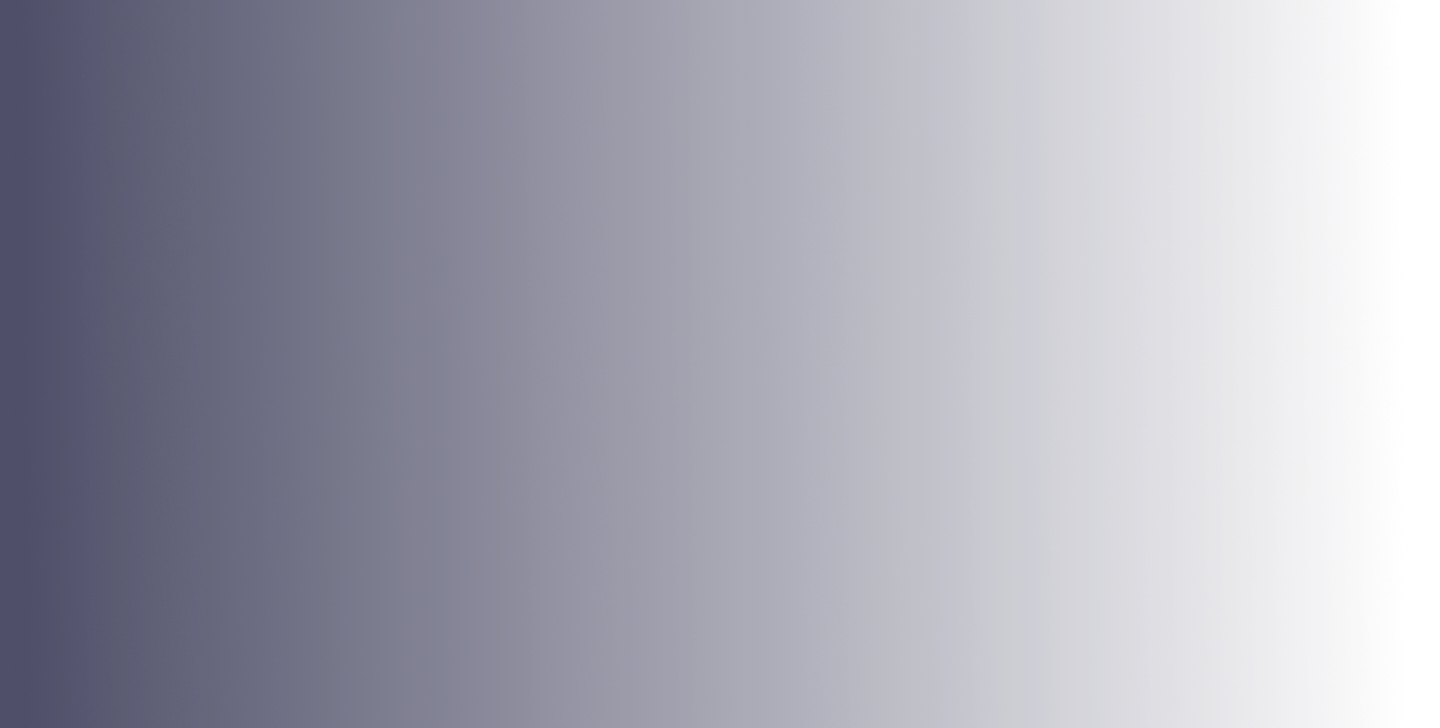

The data team at Fantix is hard at work to constantly perfect Yellowcake™ and Supernova™, two AI models that generate synthetic data. These models have been trained (and continue to learn) on real-world data. We began our journey years before ChatGPT captured the world’s attention: our models have nothing to do with LLMs, they don’t talk, they can’t draw, and they won’t get mad at you, but they are nevertheless creative and belong to a field known as GenAI for tabular data.
Synthetic data is artificially generated data that mimics real-world data. Unlike real data, which is collected from actual events, transactions, or interactions, synthetic data is created using algorithms and simulations. This data is designed to resemble the statistical properties and structure of real data, making it a valuable tool for various applications.
Synthetic data solves one of the most important business and scientific problems: lack of sufficient (real-world) data. In healthcare, synthetic data is used for research and development, allowing for the creation of realistic patient records without compromising patient privacy. Financial institutions use synthetic data to model risk, test algorithms, and develop fraud detection systems. Retailers leverage synthetic data to improve customer insights, optimize supply chains, and enhance personalized marketing strategies. Think about this the next time you get mad at your Tesla for asking you to pay attention and keep your hands on the wheel: your Model X learned on synthetic data that is crucial for training and testing autonomous driving systems, providing scenarios that might be rare or dangerous to replicate in real life.
One of the primary advantages of synthetic data is its ability to preserve privacy. Since it does not contain any real personal information, it can be used freely without risking data breaches or violating privacy regulations.
Synthetic data can be generated on-demand, providing instant access to large datasets. This is particularly useful for organizations that may not have enough real data to train machine learning models.
Collecting, cleaning, and managing real-world data can be time-consuming and expensive. Synthetic data offers a cost-effective alternative, reducing the resources needed for data collection and processing.
Real-world data often contains biases that can lead to skewed insights and unfair outcomes. Synthetic data can be designed to mitigate these biases, ensuring a more balanced and accurate dataset.